Our Technology
We've crafted a groundbreaking workflow that merges both fundamental and advanced computational biology technologies, akin to the intricate folds of an origami masterpiece. Our approach is designed to unravel the complexities of human physiology, offering unparalleled insights and solutions.
Integration of Metabolomics, Microbiome, and Metabolism Computational Modeling
Utilizing metabolomics data analysis of food and pharma products, we embark on a journey to simulate the metabolic behavior of the human microbiome. This simulation is dynamic and personalized, tailored specifically towards understanding metabolic diseases in a manner that transcends the limitations of traditional tools. Through this process, we decode the intricate metabolic changes, transforming them into expansive networks interwoven with diverse information.
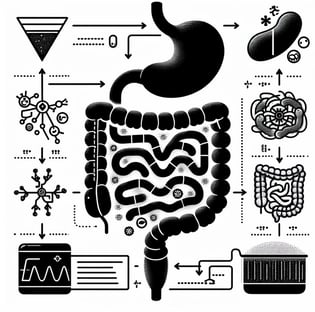
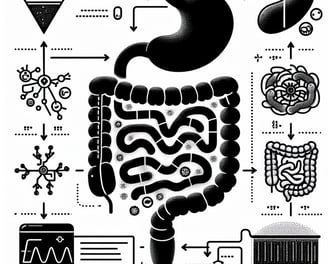
Leveraging AI and Machine Learning to Big-data Analysis and Finding Novel Insights and Ideas
The crux of our technology lies in our ability to extract specific patterns from these networks using cutting-edge machine learning tools. This enables us to unveil the therapeutic and health-oriented potential of various substances with precision and accuracy. By marrying artificial intelligence with human expertise, we deliver innovative solutions and redefine the approach to product development
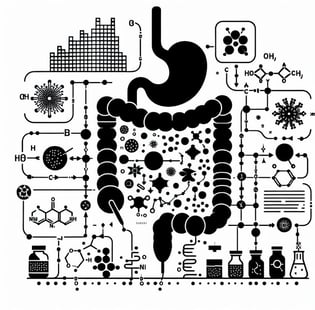
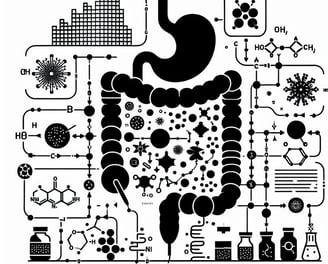
Resulting Physiological Novel Insights and Ideas
An example of the whole process:
For a clearer illustration, let's consider investigating the direct impact of a medication(drug) or nutrient or a combination of them on liver cells:
For input, we need your product's metabolomics data, so we perform a Metabolomics analysis first.
As the drug/nutrient traverses the digestive system, each segment imparts chemical changes influenced by specific conditions (e.g., pH variations, enzyme interactions). The cells within each digestive system segment actively and passively absorb substances, and the remaining components proceed to subsequent segments. This sequential process continues until completion. Notably, the immune system directly affects the digestive process. We simulate this process by computational biological systems modeling.
Next, we predict the system’s metabolic behavior by conducting drug or nutrient impacted in-silico computational metabolic models of digestive system tissue cells interconnected with microbiome and Immune system models leading to predicting metabolite secretions into the blood system. Then liver cells alterations are predicted by those metabolites.
Moreover, in the entire pathway, we implement modeling of co-cultures of active specified microbiota and relative abundances for each section: stomach microbiota, small intestine microbiota, and large intestine microbiota. The selection of the microbiome community in each section is influenced by alterations in liver disease, impacting the microbiome population. This nuanced integration allows for a more comprehensive exploration of the dynamic interplay between the digestive system, microbiota, and the development of liver diseases.
In the next step, we simulate dynamic microbiome alterations during the drug or nutrient implementation. This step unravels the microbiome community alterations and its potential biomarkers relating to healthiness and disease development or control.
The metabolic phenotypes resulting from absorption in each segment (Directly by the digestive system and indirectly by microbiome production) constitute the microenvironment of liver cells post-absorption. Liver cell metabolic models are thus exposed to these substances, facilitating investigations into metabolic changes within liver cell metabolism.
For this big-data analysis, separate physiological phenotypes are obtained for each section, and subsequent data analysis is conducted for each section. Beyond experimental examination, the results undergo AI/ML analysis, allowing the creation of diverse biological networks such as metabolic and protein-ligand networks. This approach also involves pathway analyses, establishing connections with the physiological phenotype for a comprehensive understanding of the biological interactions and responses.